Today, huge amounts of data are generated worldwide, which has led to the need for new technologies that enable the processing and analysis of these large amounts of information. In this blog post, we compare two ways of processing data: edge computing vs fog computing.
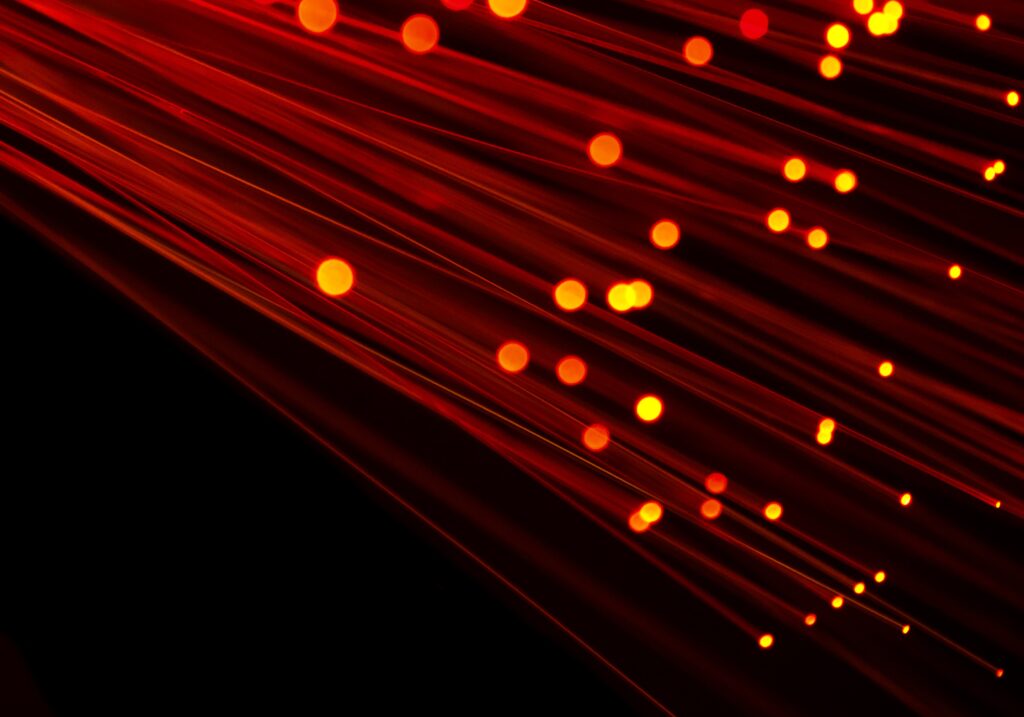
Edge computing refers to a distributed and decentralized architecture that enables data processing at the edge of the network, close to the source of the data. Edge computing offers advantages such as shorter response times, real-time data analysis and reduced network traffic and costs. You can read more about Edge computing in the post we have published on Linknovate about Edge Computing leaders and their most interesting innovations.
Another emerging technology is Fog computing, which is a network infrastructure that pre-processes data generated by IoT devices in decentralized mini data centers and sends it to the cloud. In other words, it is a horizontal architecture that shares resources and services stored anywhere in the cloud with Internet of Things devices. Fog networking offers advantages such as the option to process data in the most appropriate location, the ability to handle more data at the edge and send only the necessary data to the cloud, and the creation of network connections with a low latency period between devices, which reduces the amount of bandwidth required.
The big difference between fog and edge computing is where data processing takes place. Generally speaking, in edge computing the task is performed on the devices themselves or on a nearby device acting as an intermediary, whereas fog computing moves the activities to processors connected to the LAN or LAN hardware, so that they can be physically further away from the sensors.
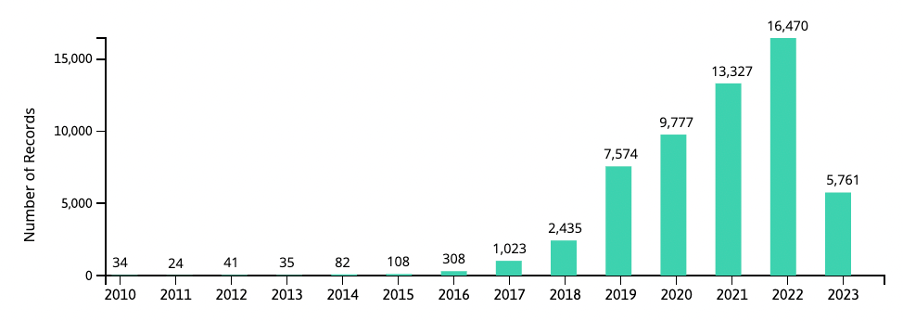
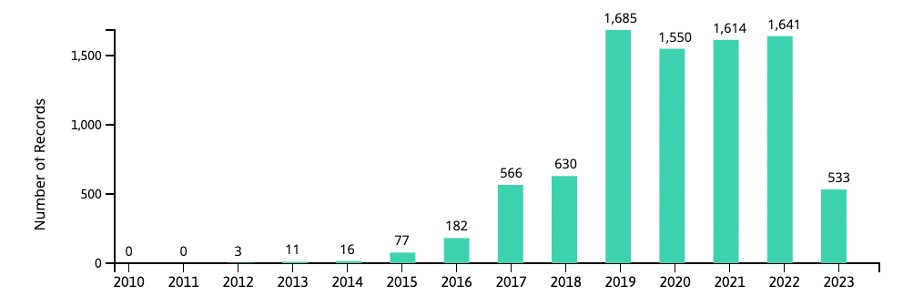
Like any other technology, edge computing and fog computing have both positive and negative aspects. To better understand them, let’s seem the advantages and disadvantages of these technologies and some of their possible applications.
Pros and cons of edge computing
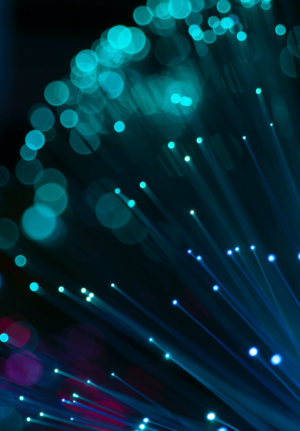
The advantages of edge computing are:
- Speed: Bringing data analysis tools and applications close to the data source reduces physical distance and data transfer time, reducing network congestion and increasing responsiveness and overall quality of service.
- Low latency: It is a key advantage, thanks to its distributed network that reduces latency and provides real-time responsiveness, keeping traffic and processing close to the application and end-user devices, leading to real-time automated decision making and improving user experience.
- Uninterrupted connections: Edge computing provides local Edge Data Centers for data storage and processing, enabling enterprises to have reliable connectivity for their IoT applications, even in the event of cloud services disruption and to operate normally under limited connectivity.
- Lower costs: Companies can reduce their costs considerably. Decrease required bandwidth. Replacing data centers with edge device solutions. Reducing data storage requirements.
Its main disadvantages:
- Complexity: The distributed system is more complex than a centralized cloud architecture, involving an heterogeneous combination of components of new technologies, some from different manufacturers and with a variety of interfaces.
- Security: IoT devices transmit trivial information and many do not have strong security measures, making them susceptible to malicious attacks.
- Less robust infrastructure: Edge data centers do not have the same infrastructure as Core Data Centers, which presents technical challenges to overcome.
Edge Computing use cases
In autonomous vehicles: Edge computing is essential for autonomous driving, as autonomous vehicles need to process large amounts of data in real time to make decisions about vehicle direction and speed. Processing data at the edge reduces latency and required bandwidth, which increases vehicle safety and improves the user experience.
Hospital patient monitoring: It can also be used in hospital patient monitoring applications, where patient sensor data is processed on nearby local devices instead of being sent to the cloud for processing. This enables faster and more efficient response in the event of a medical emergency, which can save lives.
Remote asset monitoring in the oil and gas industry: Edge computing is used for remote asset monitoring in the oil and gas industry, where data collected by sensors installed on equipment and machinery is processed at the edge before being sent to the cloud. This enables faster and more efficient asset management decisions, which can reduce operating costs and improve safety.
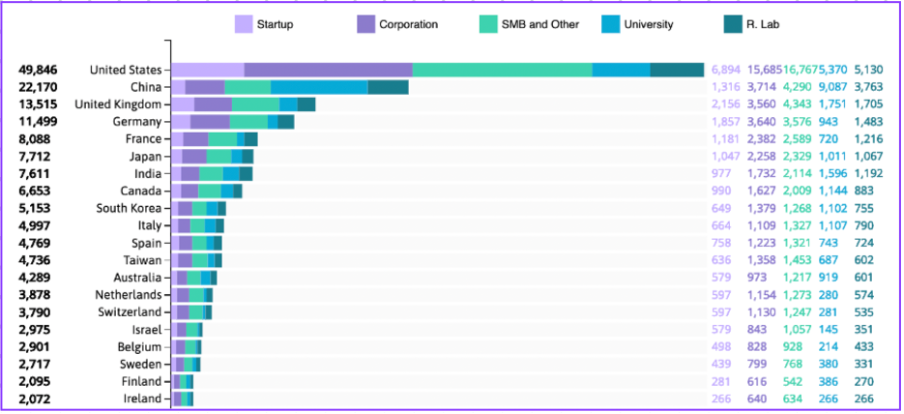
Pros and cons of fog computing
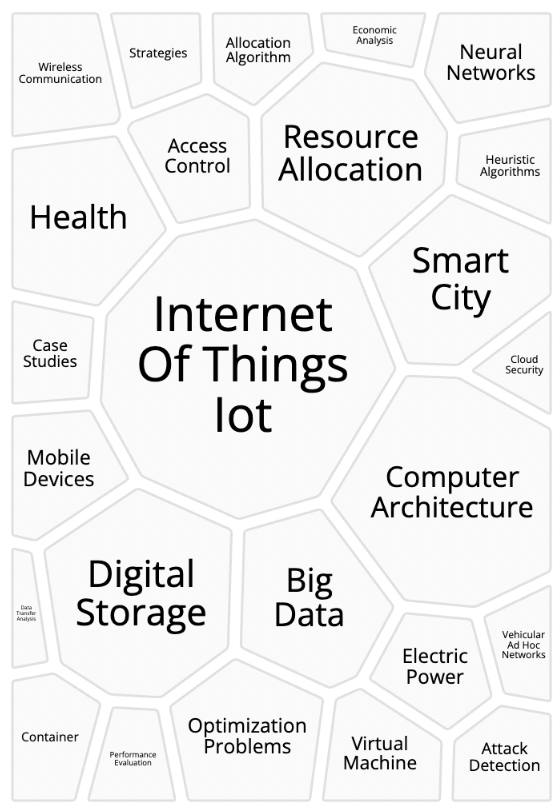
Benefits associated with fog nteworking:
- Bandwidth conservation: The volume of data sent to the cloud is reduced, thereby reducing bandwidth consumption and associated costs.
- Improved response time: Since the initial data processing occurs close to the data, latency is reduced and overall responsiveness is improved. The goal is to provide millisecond responsiveness, allowing data to be processed in near real time.
- Network-independent: Although fog computing typically places computing resources at the LAN level-rather than at the device level, as is the case with edge computing-the network could be considered part of the fog computing architecture. At the same time, however, fog computing is network-independent, in the sense that the network can be wired, Wi-Fi or even 5G.
Disadvantages of fog computing:
- Physical location: Being tied to a physical location undermines some of the advantages of cloud computing.
- Potential security issues: Under the right circumstances, fog computing can be susceptible to security issues, such as IP address spoofing or man-in-the-middle (MitM) attacks.
- Installation costs: Fog computing is a solution that uses resources both at the edge and in the cloud, which means there are associated hardware costs.
- Ambiguous concept: Although fog networking has been around for several years, its definition remains ambiguous, as different vendors define it differently.
Fog Computing use cases
Real-time health monitoring: Fog computing can be used in real-time health monitoring applications, where data collected by wearable devices (such as smartwatches or activity monitors) is processed locally on nearby devices instead of being sent to the cloud for processing. This enables faster and more efficient monitoring of a patient’s health and better medical care.
Intelligent transportation systems: Intelligent transportation systems can use fog computing to process data from sensors and cameras installed on vehicles and roads. By processing data locally on nearby devices instead of sending it to the cloud, faster and more efficient decisions can be made, such as adjusting traffic lights or rerouting traffic.
Precision agriculture: Fog computing technology can be used in precision agriculture applications, where data collected by sensors installed in fields is processed locally on nearby devices instead of being sent to the cloud for processing. This enables faster and more efficient real-time decision making on water, fertilizer and pesticide use, which can improve farming efficiency and reduce costs.
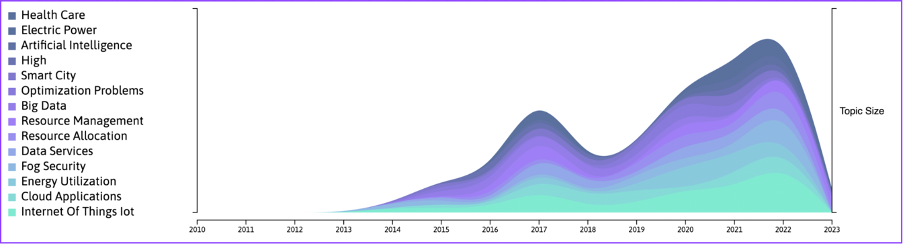
Comparing advantages and disadvantages
In summary, edge computing and fog computing are two important technologies for real-time data processing and analysis in the IoT era. The key difference between them is that edge computing processes data on the device itself, while fog networking uses processors connected to the LAN to process data physically further away from the sensors.
By choosing edge computing, you increase security and gain speed, as data does not have to travel over the network for processing. However, finding the balance between keeping data at the edge and bringing it to a central cloud when needed can be a challenge. On the other hand, in the fog model, combining multiple data sources for a more capable processing point is allowed, although this requires more infrastructure and increases costs.
In addition, data sets requiring more sophisticated algorithms are best handled in the cloud, while simpler analytical processes are best kept at the edge.